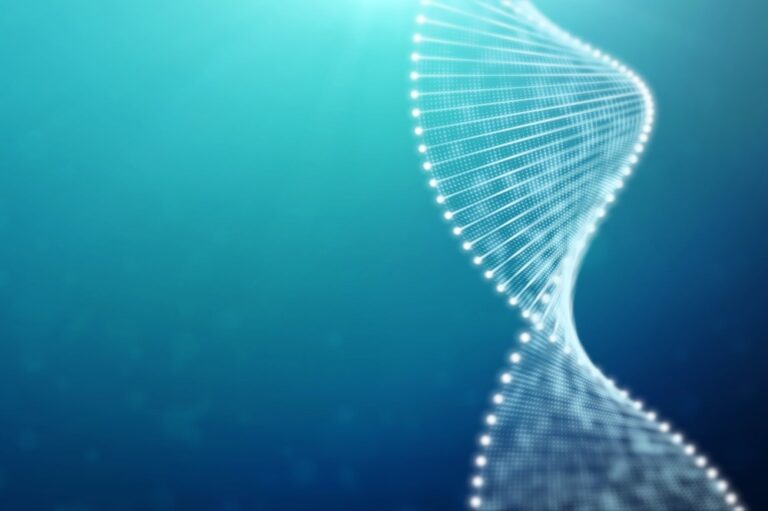
A latest study within the journal Nature Medicine analyzes longitudinal and cross-sectional changes in blood analytes related to variations in body mass index (BMI).
Study: Multiomic signatures of body mass index discover heterogeneous health phenotypes and responses to a way of life intervention. Image Credit: jivacore / Shutterstock.com
The health impacts of obesity
The prevalence of obesity has been increasing over the past 4 many years amongst adolescents, adults, and youngsters throughout the world. Several studies have reported obesity to be a significant risk factor for multiple chronic diseases akin to metabolic syndrome (MetS), type 2 diabetes mellitus (T2DM), heart problems (CVD), and certain sorts of cancer.
Even 5% weight reduction amongst obese individuals can improve metabolic and cardiovascular health, in addition to reduce the danger for obesity-related chronic diseases. Nonetheless, the physiological manifestations of obesity have been reported to differ considerably across individuals.
How is obesity measured?
Quantification of obesity takes place using the anthropometric BMI, which is body weight divided by body height squared. BMI is usually used for the first diagnosis of obesity, in addition to to evaluate the effectiveness of lifestyle interventions.
Nonetheless, there are specific limitations to using BMI as a measurement of health. For instance, BMI could cause misclassification of individuals with a high muscle-to-fat ratio as those with obesity and misjudge metabolic improvements in health post-exercise.
Omics studies have indicated that blood omic profiles can provide information on several human health conditions. A machine learning model that was trained to predict BMI through 49 BMI-associated blood metabolites was reported to supply higher obesity-related clinical measurements as in comparison with genetic predisposition for top BMI or observed BMI.
One other blood metabolomics-based model of BMI also reported differences amongst individuals with or without acute coronary syndrome. This implies that multi-omic blood profiling may also help bridge the gap between BMI and heterogeneous physiological states.
Concerning the study
The present study involved the recruitment of people that participated in a wellness program by a industrial company between 2015 and 2019. Individuals were included in the present study in the event that they were over 18 years of age, residents of any U.S. state except Recent York, and never pregnant.
Participants were included if their datasets contained all predominant omic measurements, genetic information, and a BMI measurement inside 1.5 months from the primary blood draw. The external cohort was obtained from participants who participated within the TwinsUK Registry and underwent two or more clinical visits for biological sampling between 1992 and 2022. Only participants whose datasets contained all measurements for metabolomics, obesity-related standard clinical measures and BMI were included in the present study.
Peripheral blood, saliva, and stool samples were collected from participants for evaluation of genetic ancestry, measurement of blood omics, and generation of gut microbiome data. Information on height, weight, blood pressure, waist circumference, and each day physical activity was also collected.
The evaluation of blood metabolomics, BMI, gut microbiome data, and BMI of baseline visits took place for the TwinsUK participants. Machine learning models were trained to predict baseline BMI for every of the omics platforms including proteomics, metabolomics, and clinical lab, or together with clinical labs (chemistries)-based BMI (ChemBMI), proteomics-based BMI (ProtBMI), metabolomics-based BMI (MetBMI), and combined omics-based BMI (CombiBMI) models. One other ten fitted sparse models were generated using the least absolute shrinkage and selection operator (LASSO) algorithm for every omics category.
This was followed by the health classification of every participant based on the World Health Organization (WHO) international standards for BMI cutoffs. Gut microbiome models were also generated for the classification of obesity. Assessments of longitudinal changes took place within the measured and omics-inferred BMIs. Finally, an evaluation of the plasma analyte correlation network was performed.
Study findings
A complete of 1,277 adults participated within the study, most of whom were White, female, and middle-aged. The BMI prevalence at baseline was similar amongst the traditional, obese, and obese classes.
The models retained 30 proteins, 62 metabolites, 20 clinical laboratory tests, in addition to 132 analytes. The CombiBMI model was found to be the perfect in BMI prediction.
Investigation of the TwinsUK cohort indicated that blood metabolomics higher captures BMI as compared to straightforward clinical measures. Notably, omics-inferred BMI maintained the characteristics of classical BMI.
Proteins were the strongest predictors within the CombiBMI model. More specifically, fatty acid-binding protein 4 (FABP4), adrenomedullin (ADM), and leptin (LEP) were positive regulators, while advanced glycosylation end-product-specific receptor (AGER) and insulin-like growth factor-binding protein 1 (IGFBP1) were negative regulators.
The misclassification rate of omics-inferred BMI was about 30% across all BMI classes and omics categories. The mismatched groups of the traditional BMI class showed higher values of the markers positively related to BMI and lower values of the markers negatively related to BMI, while the alternative was observed for the mismatched group of the obese BMI class. The omics-based BMI model also captured obesity characteristics, including abdominal obesity.
The MetBMI class reflected bacterial diversity higher than the usual BMI class and had stronger associations with gut microbiome features. Lifestyle interventions decreased the general BMI estimate of the whole cohort, where a decrease of MetBMI was the best and ProtBMI was the least.
A complete of 100 analyte–analyte correlation pairs were significantly modified by the baseline MetBMI. Amongst them, 27 analyte-analyte correlation pairs were significantly modified by days in this system and were mainly derived from metabolites.
One such time-varying pair was homoarginine and phenyllactate (PLA). A positive association between homoarginine and PLA was observed within the obese MetBMI class at baseline, which became weaker in the course of the intervention.
Conclusions
The present study demonstrates the importance of blood multi-omic profiling for preventive and predictive medicine. Moreover, these findings display that multi-omic characterization of obesity may be useful for the characterization of metabolic health, in addition to identifying targets for health management.
Limitations
The study has certain limitations. First, the analytes retained within the omics-based models won’t have causal relationships with obesity phenotypes. Second, the study didn’t measure all biomolecules in blood.
A further limitation is that the present study was unable to guage the effectiveness of the life-style intervention. The study findings are also not generalizable.
Journal reference:
- Watanabe, K., Wilmanski, T., Diener, C., et al. (2023). Multiomic signatures of body mass index discover heterogeneous health phenotypes and responses to a way of life intervention. Nature Medicine. doi:10.1038/s41591-023-02248-0.