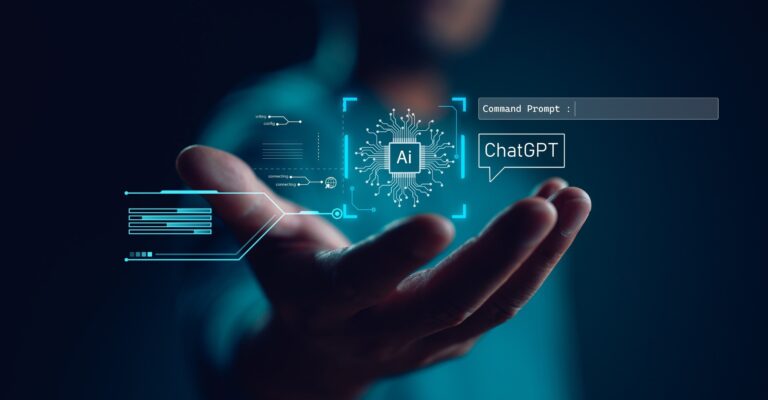
Amongst all neurological diseases, the incidence of Parkinson’s disease (PD) has increased significantly. PD is often diagnosed on the idea of motor nerve symptoms, equivalent to resting tremors, rigidity, and bradykinesia. Nonetheless, the detection of non-motor symptoms, equivalent to constipation, apathy, lack of smell, and sleep disorders, could assist in the early diagnosis of PD by several years to many years.
In a recent ACS Central Science study, scientists from the University of Recent South Wales (UNSW) discuss a machine learning (ML)-based tool that may detect PD years before the primary onset of symptoms.
Study: Interpretable Machine Learning on Metabolomics Data Reveals Biomarkers for Parkinson’s Disease. Image Credit: SomYuZu / Shutterstock.com
Background
At present, the general diagnostic accuracy for PD based on motor symptoms is 80%. This accuracy might be increased if PD was diagnosed based on biomarkers relatively than primarily depending on physical symptoms.
Several diseases are detected based on biomarkers related to metabolic processes. Biometabolites from blood plasma or serum samples are assessed using analytical tools equivalent to mass spectrometry (MS).
Non-invasive diagnostic methods using skin sebum and breath have recently gained popularity. Previous studies have shown that MS can project differential metabolite profiles between pre-PD candidates and healthy individuals.
This difference in metabolite profiles was observed as much as 15 years prior to a clinical diagnosis of PD. Thus, metabolite biomarkers might be used to detect PD much sooner than recently used approaches.
ML approaches are widely used to develop accurate prediction models for disease diagnosis using large metabolomics data. Nonetheless, the event of prediction models based on whole metabolomics data sets is related to many disadvantages, including overtraining that might reduce diagnostic performance. The vast majority of models are developed using a smaller subset of features, that are pre-determined by traditional statistical methods.
Some ML approaches, equivalent to a linear support vector machine (SVM) and partial least-squares-discriminant evaluation (PLSDA) can fail to account for key features in metabolomics data sets. Nonetheless, this limitation was resolved by advanced ML methods, equivalent to neural networks (NN), which have been particularly designed for processing large data.
NN is used to develop models which have a non-linear effect. A key drawback of NN-based predictive models is the shortage of mechanistic information and uninterpretable models.
Shapley additive explanations (SHAP) have recently been developed to interpret ML models. Nonetheless, this system has not yet been used to research metabolomics data sets.
In regards to the study
In the present study, researchers evaluated blood samples obtained from the Spanish European Prospective Study on Nutrition and Cancer (EPIC) using different analytical tools equivalent to gas chromatography-MS (GC-MS), capillary electrophoresis-MS (CE-MS) and liquid chromatography-MS (LC-MS).
The EPIC study provided metabolomics data from blood plasma samples obtained from each healthy candidates, in addition to those that later developed PD as much as 15 years later after their sample was originally collected.
Diane Zhang, a researcher at UNSW, developed an ML tool called Classification and Rating Evaluation using Neural Networks generates Knowledge from MS (CRANK-MS). This tool was built to interpret the NN-based framework to research the metabolomics dataset generated by the analytical tools.
CRANK-MS is comprised of several features, including integrated model parameters that provide high dimensionality of metabolomics data sets to be analyzed without requiring any preselecting chemical features.
CRANK-MS also includes SHAP to retrospectively explore and discover key chemical features that assist in accurate model prediction. Furthermore, SHAP enables benchmark testing with five well-known ML methods to match diagnostic performance and validate chemical features.
The metabolomic data obtained from 39 patients who developed PD as much as 15 years later were investigated through the newly developed ML-based tool. The metabolite profile of 39 pre-PD patients was compared with 39 matched control patients, which provided a novel combination of metabolites that might be used as an early warning sign for PD incidence. Notably, this ML approach exhibited a better accuracy for predicting PD upfront of clinical diagnosis.
Five metabolites scored consistently high across all six ML models, thus indicating their potential utility for predicting the long run development of PD. These metabolites’ classes included polyfluorinated alkyl substance (PFAS), triterpenoid, diacylglycerol, steroid, and cholestane steroid.
The detected diacylglycerol metabolite 1,2-diacylglycerol (34:2) isomers are certain vegetable oils like olive oil, which is regularly consumed within the Mediterranean weight loss plan. PFAS is an environmental neurotoxin that may alter neuronal cell processing, signaling, and performance. Thus, each dietary and environmental aspects may contribute to the event of PD.
Conclusions
CRANK-MS is publicly available to all researchers fascinated about disease diagnosis using the ML approach based on metabolomic data.
The appliance of CRANK-MS to detect Parkinson’s disease is only one example of how AI can improve the way in which we diagnose and monitor diseases. What’s exciting is that CRANK-MS may be readily applied to other diseases to discover recent biomarkers of interest. She further claimed that this tool is user-friendly and might generate results “in lower than 10 minutes on a standard laptop.”
Journal reference:
- Zhang, D. J., Xue, C., Kolachalama, V. B., & Donald, W. A. (2023) Interpretable Machine Learning on Metabolomics Data Reveals Biomarkers for Parkinson’s Disease. ACS Central Science. doi:10.1021/acscentsci.2c01468