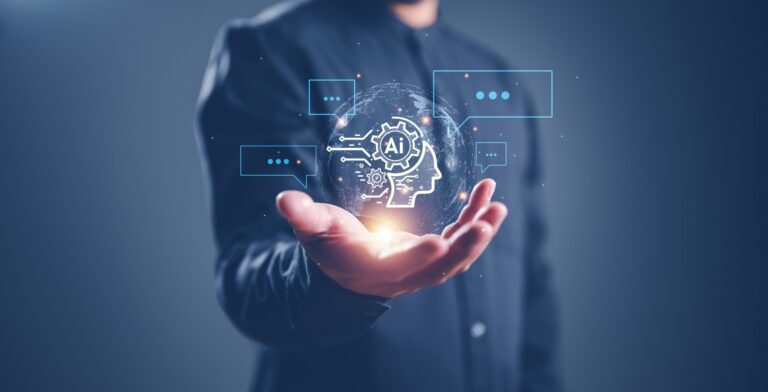
In a recent review published in Science, researchers discussed the role of artificial intelligence (AI) in stopping outbreaks of infectious diseases and future pandemics.
Study: Leveraging artificial intelligence within the fight against infectious diseases. Image Credit: SomYuZu/Shutterstock.com
Background
Despite developments in molecular genetics, computers, and pharmaceutical chemistry, infectious diseases remain a serious global health problem.
Multidisciplinary cooperation might be required to deal with the difficulties posed by disease outbreaks, pandemics, and antibiotic resistance.
Together with synthetic and systems biology, AI is accelerating progress, increasing anti-infective medication discovery, improving our comprehension of infection biology, and expediting diagnostic research.
In regards to the review
In the current review, researchers presented the challenges in stopping infectious illnesses and the contribution of AI to disease prevention.
Global Challenges in stopping infectious diseases
Challenges in understanding the biological mechanisms underlying disease and developing infection prevention measures are essential in managing outbreaks and recent pathogens like severe acute respiratory syndrome coronavirus 2 (SARS-CoV-2), monkeypox, Ebola, H5N1 influenza, Marburg virus, Zika, measles, MERS, and Escherichia coli.
Problematic pathogenic organisms include methicillin-resistant Staphylococcus aureus (MRSA), carbapenem-resistant Enterobacteriaceae (CRE), vancomycin-resistant Enterococcus (VRE), multidrug-resistant tuberculosis (MDR-TB), extended-spectrum β-lactamase (ESBL)-secreting bacterial organisms, and protracted pathogens reminiscent of Neisseria gonorrhoeae, Candida auris, T. gondii, and P. falciparum.
Antimicrobial stewardship, developing novel anti-infective medications, and understanding their mechanisms of motion are crucial to combat these challenges.
Moreover, developing low-cost and field-deployable diagnostics, improving test accuracy, detecting antimicrobial resistance, and making effective disease treatments available are essential for addressing persistent and neglected infections [such as Lyme disease, chronic hepatitis B virus (HBV) and hepatitis C virus (HCV) infections, chronic mycotic infections, human immunodeficiency virus (HIV)-caused acquired immunodeficiency syndrome (AIDS), and those among individuals with poor access to health resources].
Artificial intelligence and machine learning for stopping infections
AI-based approaches have the potential to integrate large amounts of quantitative and omics data, making them particularly adept at coping with biological complexity.
Machine learning (ML), a subcategory of artificial intelligence, uses data to coach machines to make predictions and has helped facilitate searches of small-molecule databases.
ML approaches include supervised graph neural networks and unsupervised generative models. Supervised machine learning algorithms examine structured and unstructured glycan, protein, nucleic acid, and cell phenotypic information to uncover vital characteristics and molecular structures that regulate interactions between hosts, pathogens, and immune system responses.
Inverse vaccinology, which predicts antigens based on immunological and genetic data, has been aided by supervised ML techniques like Vaxign-ML.
De novo chemical arrangements and peptide chains are proposed using generative ML models, which could also be produced and assessed. Drug development will also be aided by generative systems reminiscent of GPT-4 and NVIDIA’s BioNeMo, which integrate different scientific data streams to enhance our comprehension of the basic biological and chemical dynamics.
AI can predict anti-infective medication activity, drug-target interactions, and therapeutic design. ML approaches to anti-infective drug discovery have centered on training models to discover recent drugs or uses of currently used drugs. A key good thing about ML approaches is that they will virtually screen compound libraries at a scale (>109 compounds) that will be unimaginable to screen empirically.
AI approaches relevant to anti-infective drug discovery include inputs reminiscent of phenotypic screens, target-specific screens, and anti-infective susceptibilities; models including graph neural networks, random forest classifiers, and explainable models; and outputs reminiscent of growth inhibition, antimicrobial activity, and target-binding activity.
Recent advancements in merging artificial intelligence with artificial biology, genetic expression evaluation, imaging, and mass spectrometry have significantly increased our capability to discover infections and predict medication resistance.
AI models are used for gene expression-, mass spectrometry-, and imaging-based diagnostics, and AST stays vital for informing the usage of anti-infective drugs. AI can elucidate infection biology, facilitate vaccine design, and inform anti-infective treatment strategies.
Infection biology inputs include macromolecular sequences, protein structures, microscopy, and morphology; models include network modeling, interaction modeling, and language modeling; and outputs include immunogenicity, inter-protein interactions, and pathogen killing and escape.
For vaccine design, AI inputs include nucleic acid or protein sequences, protein structures, and antigen-binding information; models include sequence-to-function-type models, classifier ensembles, and neural networks; and outputs include antigen presentation, vaccine efficacy, and translational efficacy.
Resulting from the strong programmability of biological components, the regular synthesis of massive or sequence-based information sets, and the capability of ML to retrieve pertinent data from biological and molecular systems in disease biological sciences, AI can aid synthetic biology studies and the event of diagnostics.
Conclusion
Based on the review findings, ML and AI have revolutionized infectious disease research by analyzing large datasets and providing useful insights. Nevertheless, challenges in diagnosis include low data quality, limited generalizability, and high diagnostic predictions.
Experiments involving large datasets and comprehensive benchmarking datasets are required to enhance ML models.
Multi-dimensional drug interaction prediction can enhance treatment options, forecast unwanted side effects, and boost the success rates of novel medications in clinical research.